[Work in Progress]
Today there are predictions of Singularity event happening in 2040s wherein robots would be overtaking the humans in intelligence as well as in speech and locomotion. Would this event really signify robots acquiring intelligence as we humans understand it?
This post is triggered by Chomsky’s critique of data scientists adaptation of AI as exemplified in the recent Chomsky vs Norvig (CTO of Google) debate [1],[2]. It is also motivated by the prediction that there would be no need for any theory building and testing by humans [3].
AI vs Data Sciences: Chomsky’s Cognitive Science vs Skinner’s Behavioral Sciences
- Presented at ICOSST 2017, KICS UET Lahore, http://icosst.kics.edu.pk/2017/
- A modified form of this post was also presented as a keynote speech at the 2nd International Conference on Computer and Information Sciences at PAF KIET on March 26, 2018]
Who is Noam Chomsky
His ideas have found a wide application in diverse fields and can effortlessly switch across different fields.
Who is BF Skinner
"Skinner considered free will an illusion and human action dependent on consequences of previous actions. If the consequences are bad, there is a high chance the action will not be repeated; if the consequences are good, the probability of the action being repeated become stronger.[7] Skinner called this the principle of reinforcement. To strengthen behavior, Skinner used operant conditioning, and he considered the rate of response to be the most effective measure of response strength.Skinner developed behavior analysis, the philosophy of that science he called radical behaviorism,[12] and founded a school of experimental research psychology—the experimental analysis of behavior. He imagined the application of his ideas to the design of a human community in his utopian novel, Walden Two,[13] and his analysis of human behavior culminated in his work, Verbal Behavior.[14] Skinner was a prolific author who published 21 books and 180 articles.[15][16] Contemporary academia considers Skinner a pioneer of modern behaviorism, along with John B. Watson and Ivan Pavlov. A June 2002 survey listed Skinner as the most influential psychologist of the 20th century. " [From Wikipedia]
Why they are important
Chomsky vs Skinner
Chomsky’s Cognitive Psychology
- •Intrinsic Motivation
- Remove external demotivators- Demming
- Natural creativity
- Self-learning, self-expression
- Internal Human Mind Working
- Language ability
- Impulse; Not Habit
- Cognitive Processor
- Output is function of input, internal representations
- Compiler program structures
BF Skinner’s Behavioral Psychology
- • Extrinsic Motivation
- Carrot and stick
- Conditioning
- Behavioral control
- Non Human Organism Modes
- Pigeons, rats, dogs
- Habit
- Behavior modification
- Output is function of input
- Based on history
Chomsky vs Skinner
Chomsky’s Language Conception
- Complex internal representations
- Encoding in genome
- Maturation with right data into complex computational system
- Cannot be usefully broken down into a set of associations
- Language faculty
- A genetic endowment like visual, immune, circulatory systems
- Approach: Similar to other more down-to-earth biological systems
BF Skinner’s Behaviorist Convention
- Historical associations
- Stimulus => animal's response
- Empirical statistical analysis
- Predicting future
- as a function of the past
- Behaviorist associations fail to explain
- Richness of linguistic knowledge
- Endless creative use of language
- How children acquire it with exposure to only minimal language in environment
Impact of Behavioral Psychology
- 20th Century management
- Org behavior, HRM, Control, monitoring
- 20th Century schooling
- Text books, curriculum, grading, pedagogy
- Problems:
- Value of book is inversely proportional to count of the word behavior occurs in the book Alfie Kohn
- Lack of creativity, innovation
- Dumbing of students, employees
According to Chomsky:
Data Science with heavy use of statistical techniques to identify patterns in big data will not yield explanatory intelligence related scientific insights.Eg. Google search "physicist Sir Isaac Newton"
New AI is unlikely to yield
“general principles about the nature of intelligent beings or about cognition”Issues
- Why learn anything if you can do a lookup!
- Is human understanding necessary for making successful predictions?
- If “no,” then predictions are best made by churning mountains of data through powerful algorithms
- Role of scientist may fundamentally change forever.
- AIs attempt to use data science is like
- Students googling answers to math homework
- Will such answers serve them well in the long term.
- AI algorithms can successfully predict planets’ motion
- without ever discovering Kepler’s laws,
- Google can store all recorded positions of stars, planets in big data.
- Is science more than the accumulation of facts, producing predictions?
Fundamental Issues
- Why vs How
- – If we know “how”, why we want to know “why”?
- – “Closing of American Mind”
- – Simon Sinek ted talk on how leaders inspire
- Death of theorizing? Death of scientific method
- – Who needs theory, if the data can itself generate conjecture, and can itself statistically prove/disprove through statistical analysis of big data
- Just because modeling internals of mind is difficult, we should find a work around!
References Links to be Added
[1] Chomsky on AI vs data science:
Noam Chomsky on Where Artificial Intelligence Went Wrong
https://www.theatlantic.com/technology/archive/2012/11/noam-chomsky-on-where-artificial-intelligence-went-wrong/261637/An extended conversation with the legendary linguist
By Yarden Katz
NOVEMBER 1, 2012
If one were to rank a list of civilization's greatest and most elusive intellectual challenges, the problem of "decoding" ourselves—understanding the inner workings of our minds and our brains, and how the architecture of these elements is encoded in our genome—would surely be at the top. Yet the diverse fields that took on this challenge, from philosophy and psychology to computer science and neuroscience, have been fraught with disagreement about the right approach.
In 1956, the computer scientist John McCarthy coined the term "Artificial Intelligence" (AI) to describe the study of intelligence by implementing its essential features on a computer. Instantiating an intelligent system using man-made hardware, rather than our own "biological hardware" of cells and tissues, would show ultimate understanding, and have obvious practical applications in the creation of intelligent devices or even robots.Some of McCarthy's colleagues in neighboring departments, however, were more interested in how intelligence is implemented in humans (and other animals) first. Noam Chomsky and others worked on what became cognitive science, a field aimed at uncovering the mental representations and rules that underlie our perceptual and cognitive abilities. Chomsky and his colleagues had to overthrow the then-dominant paradigm of behaviorism, championed by Harvard psychologist B.F. Skinner, where animal behavior was reduced to a simple set of associations between an action and its subsequent reward or punishment. The undoing of Skinner's grip on psychology is commonly marked by Chomsky's 1959 critical review of Skinner's book Verbal Behavior, a book in which Skinner attempted to explain linguistic ability using behaviorist principles.
Skinner's approach stressed the historical associations between a stimulus and the animal's response—an approach easily framed as a kind of empirical statistical analysis, predicting the future as a function of the past. Chomsky's conception of language, on the other hand, stressed the complexity of internal representations, encoded in the genome, and their maturation in light of the right data into a sophisticated computational system, one that cannot be usefully broken down into a set of associations. Behaviorist principles of associations could not explain the richness of linguistic knowledge, our endlessly creative use of it, or how quickly children acquire it with only minimal and imperfect exposure to language presented by their environment. The "language faculty," as Chomsky referred to it, was part of the organism's genetic endowment, much like the visual system, the immune system and the circulatory system, and we ought to approach it just as we approach these other more down-to-earth biological systems.
David Marr, a neuroscientist colleague of Chomsky's at MIT, defined a general framework for studying complex biological systems (like the brain) in his influential book Vision, one that Chomsky's analysis of the language capacity more or less fits into. According to Marr, a complex biological system can be understood at three distinct levels. The first level ("computational level") describes the input and output to the system, which define the task the system is performing. In the case of the visual system, the input might be the image projected on our retina and the output might our brain's identification of the objects present in the image we had observed. The second level ("algorithmic level") describes the procedure by which an input is converted to an output, i.e. how the image on our retina can be processed to achieve the task described by the computational level. Finally, the third level ("implementation level") describes how our own biological hardware of cells implements the procedure described by the algorithmic level.
The approach taken by Chomsky and Marr toward understanding how our minds achieve what they do is as different as can be from behaviorism. The emphasis here is on the internal structure of the system that enables it to perform a task, rather than on external association between past behavior of the system and the environment. The goal is to dig into the "black box" that drives the system and describe its inner workings, much like how a computer scientist would explain how a cleverly designed piece of software works and how it can be executed on a desktop computer.
As written today, the history of cognitive science is a story of the unequivocal triumph of an essentially Chomskyian approach over Skinner's behaviorist paradigm—an achievement commonly referred to as the "cognitive revolution," though Chomsky himself rejects this term. While this may be a relatively accurate depiction in cognitive science and psychology, behaviorist thinking is far from dead in related disciplines. Behaviorist experimental paradigms and associationist explanations for animal behavior are used routinely by neuroscientists who aim to study the neurobiology of behavior in laboratory animals such as rodents, where the systematic three-level framework advocated by Marr is not applied.
In May of last year, during the 150th anniversary of the Massachusetts Institute of Technology, a symposium on "Brains, Minds and Machines" took place, where leading computer scientists, psychologists and neuroscientists gathered to discuss the past and future of artificial intelligence and its connection to the neurosciences.
The gathering was meant to inspire multidisciplinary enthusiasm for the revival of the scientific question from which the field of artificial intelligence originated: How does intelligence work? How does our brain give rise to our cognitive abilities, and could this ever be implemented in a machine?
Noam Chomsky, speaking in the symposium, wasn't so enthused. Chomsky critiqued the field of AI for adopting an approach reminiscent of behaviorism, except in more modern, computationally sophisticated form. Chomsky argued that the field's heavy use of statistical techniques to pick regularities in masses of data is unlikely to yield the explanatory insight that science ought to offer. For Chomsky, the "new AI"—focused on using statistical learning techniques to better mine and predict data— is unlikely to yield general principles about the nature of intelligent beings or about cognition.
This critique sparked an elaborate reply to Chomsky from Google's director of research and noted AI researcher, Peter Norvig, who defended the use of statistical models and argued that AI's new methods and definition of progress is not far off from what happens in the other sciences.
Chomsky acknowledged that the statistical approach might have practical value, just as in the example of a useful search engine, and is enabled by the advent of fast computers capable of processing massive data. But as far as a science goes, Chomsky would argue it is inadequate, or more harshly, kind of shallow. We wouldn't have taught the computer much about what the phrase "physicist Sir Isaac Newton" really means, even if we can build a search engine that returns sensible hits to users who type the phrase in.
It turns out that related disagreements have been pressing biologists who try to understand more traditional biological systems of the sort Chomsky likened to the language faculty. Just as the computing revolution enabled the massive data analysis that fuels the "new AI," so has the sequencing revolution in modern biology given rise to the blooming fields of genomics and systems biology. High-throughput sequencing, a technique by which millions of DNA molecules can be read quickly and cheaply, turned the sequencing of a genome from a decade-long expensive venture to an affordable, commonplace laboratory procedure. Rather than painstakingly studying genes in isolation, we can now observe the behavior of a system of genes acting in cells as a whole, in hundreds or thousands of different conditions.
The sequencing revolution has just begun and a staggering amount of data has already been obtained, bringing with it much promise and hype for new therapeutics and diagnoses for human disease. For example, when a conventional cancer drug fails to work for a group of patients, the answer might lie in the genome of the patients, which might have a special property that prevents the drug from acting. With enough data comparing the relevant features of genomes from these cancer patients and the right control groups, custom-made drugs might be discovered, leading to a kind of "personalized medicine." Implicit in this endeavor is the assumption that with enough sophisticated statistical tools and a large enough collection of data, signals of interest can be weeded it out from the noise in large and poorly understood biological systems.
The success of fields like personalized medicine and other offshoots of the sequencing revolution and the systems-biology approach hinge upon our ability to deal with what Chomsky called "masses of unanalyzed data"—placing biology in the center of a debate similar to the one taking place in psychology and artificial intelligence since the 1960s.
Systems biology did not rise without skepticism. The great geneticist and Nobel-prize winning biologist Sydney Brenner once defined the field as "low input, high throughput, no output science." Brenner, a contemporary of Chomsky who also participated in the same symposium on AI, was equally skeptical about new systems approaches to understanding the brain. When describing an up-and-coming systems approach to mapping brain circuits called Connectomics, which seeks to map the wiring of all neurons in the brain (i.e. diagramming which nerve cells are connected to others), Brenner called it a "form of insanity."
Brenner's catch-phrase bite at systems biology and related techniques in neuroscience is not far off from Chomsky's criticism of AI. An unlikely pair, systems biology and artificial intelligence both face the same fundamental task of reverse-engineering a highly complex system whose inner workings are largely a mystery. Yet, ever-improving technologies yield massive data related to the system, only a fraction of which might be relevant. Do we rely on powerful computing and statistical approaches to tease apart signal from noise, or do we look for the more basic principles that underlie the system and explain its essence? The urge to gather more data is irresistible, though it's not always clear what theoretical framework these data might fit into. These debates raise an old and general question in the philosophy of science: What makes a satisfying scientific theory or explanation, and how ought success be defined for science?
I sat with Noam Chomsky on an April afternoon in a somewhat disheveled conference room, tucked in a hidden corner of Frank Gehry's dazzling Stata Center at MIT. I wanted to better understand Chomsky's critique of artificial intelligence and why it may be headed in the wrong direction. I also wanted to explore the implications of this critique for other branches of science, such neuroscience and systems biology, which all face the challenge of reverse-engineering complex systems—and where researchers often find themselves in an ever-expanding sea of massive data. The motivation for the interview was in part that Chomsky is rarely asked about scientific topics nowadays. Journalists are too occupied with getting his views on U.S. foreign policy, the Middle East, the Obama administration and other standard topics. Another reason was that Chomsky belongs to a rare and special breed of intellectuals, one that is quickly becoming extinct. Ever since Isaiah Berlin's famous essay, it has become a favorite pastime of academics to place various thinkers and scientists on the "Hedgehog-Fox" continuum: the Hedgehog, a meticulous and specialized worker, driven by incremental progress in a clearly defined field versus the Fox, a flashier, ideas-driven thinker who jumps from question to question, ignoring field boundaries and applying his or her skills where they seem applicable. Chomsky is special because he makes this distinction seem like a tired old cliche. Chomsky's depth doesn't come at the expense of versatility or breadth, yet for the most part, he devoted his entire scientific career to the study of defined topics in linguistics and cognitive science. Chomsky's work has had tremendous influence on a variety of fields outside his own, including computer science and philosophy, and he has not shied away from discussing and critiquing the influence of these ideas, making him a particularly interesting person to interview. Videos of the interview can be found here.
I want to start with a very basic question. At the beginning of AI, people were extremely optimistic about the field's progress, but it hasn't turned out that way. Why has it been so difficult? If you ask neuroscientists why understanding the brain is so difficult, they give you very intellectually unsatisfying answers, like that the brain has billions of cells, and we can't record from all of them, and so on.
Chomsky: There's something to that. If you take a look at the progress of science, the sciences are kind of a continuum, but they're broken up into fields. The greatest progress is in the sciences that study the simplest systems. So take, say physics—greatest progress there. But one of the reasons is that the physicists have an advantage that no other branch of sciences has. If something gets too complicated, they hand it to someone else.
Like the chemists?
Chomsky: If a molecule is too big, you give it to the chemists. The chemists, for them, if the molecule is too big or the system gets too big, you give it to the biologists. And if it gets too big for them, they give it to the psychologists, and finally it ends up in the hands of the literary critic, and so on. So what the neuroscientists are saying is not completely false.
However, it could be—and it has been argued in my view rather plausibly, though neuroscientists don't like it—that neuroscience for the last couple hundred years has been on the wrong track. There's a fairly recent book by a very good cognitive neuroscientist, Randy Gallistel and King, arguing—in my view, plausibly—that neuroscience developed kind of enthralled to associationism and related views of the way humans and animals work. And as a result they've been looking for things that have the properties of associationist psychology."It could be—and it has been argued, in my view rather plausibly, though neuroscientists don't like it—that neuroscience for the last couple hundred years has been on the wrong track."
Like Hebbian plasticity? [Editor's note: A theory, attributed to Donald Hebb, that associations between an environmental stimulus and a response to the stimulus can be encoded by strengthening of synaptic connections between neurons.]
Chomsky: Well, like strengthening synaptic connections. Gallistel has been arguing for years that if you want to study the brain properly you should begin, kind of like Marr, by asking what tasks is it performing. So he's mostly interested in insects. So if you want to study, say, the neurology of an ant, you ask what does the ant do? It turns out the ants do pretty complicated things, like path integration, for example. If you look at bees, bee navigation involves quite complicated computations, involving position of the sun, and so on and so forth. But in general what he argues is that if you take a look at animal cognition, human too, it's computational systems. Therefore, you want to look the units of computation. Think about a Turing machine, say, which is the simplest form of computation, you have to find units that have properties like "read," "write," and "address." That's the minimal computational unit, so you got to look in the brain for those. You're never going to find them if you look for strengthening of synaptic connections or field properties, and so on. You've got to start by looking for what's there and what's working and you see that from Marr's highest level.
Right, but most neuroscientists do not sit down and describe the inputs and outputs to the problem that they're studying. They're more driven by say, putting a mouse in a learning task and recording as many neurons possible, or asking if Gene X is required for the learning task, and so on. These are the kinds of statements that their experiments generate.
Chomsky: That's right..
Is that conceptually flawed?
Chomsky: Well, you know, you may get useful information from it. But if what's actually going on is some kind of computation involving computational units, you're not going to find them that way. It's kind of, looking at the wrong lamp post, sort of. It's a debate ... I don't think Gallistel's position is very widely accepted among neuroscientists, but it's not an implausible position, and it's basically in the spirit of Marr's analysis. So when you're studying vision, he argues, you first ask what kind of computational tasks is the visual system carrying out. And then you look for an algorithm that might carry out those computations and finally you search for mechanisms of the kind that would make the algorithm work. Otherwise, you may never find anything. There are many examples of this, even in the hard sciences, but certainly in the soft sciences. People tend to study what you know how to study, I mean that makes sense. You have certain experimental techniques, you have certain level of understanding, you try to push the envelope—which is okay, I mean, it's not a criticism, but people do what you can do. On the other hand, it's worth thinking whether you're aiming in the right direction. And it could be that if you take roughly the Marr-Gallistel point of view, which personally I'm sympathetic to, you would work differently, look for different kind of experiments."Neuroscience developed kind of enthralled to associationism and related views of the way humans and animals work. And as a result they've been looking for things that have the properties of associationist psychology."
Right, so I think a key idea in Marr is, like you said, finding the right units to describing the problem, sort of the right "level of abstraction" if you will. So if we take a concrete example of a new field in neuroscience, called Connectomics, where the goal is to find the wiring diagram of very complex organisms, find the connectivity of all the neurons in say human cerebral cortex, or mouse cortex. This approach was criticized by Sidney Brenner, who in many ways is [historically] one of the originators of the approach. Advocates of this field don't stop to ask if the wiring diagram is the right level of abstraction—maybe it's not, so what is your view on that?
Chomsky: Well, there are much simpler questions. Like here at MIT, there's been an interdisciplinary program on the nematode C. elegans for decades, and as far as I understand, even with this miniscule animal, where you know the wiring diagram, I think there's 800 neurons or something ...
I think 300..
Chomsky: … Still, you can't predict what the thing [C. elegans nematode] is going to do. Maybe because you're looking in the wrong place.
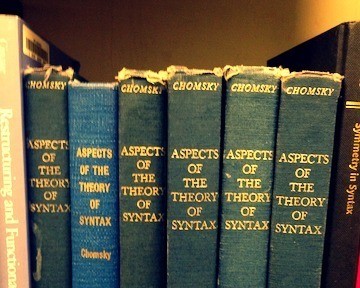
I'd like to shift the topic to different methodologies that were used in AI. So "Good Old Fashioned AI," as it's labeled now, made strong use of formalisms in the tradition of Gottlob Frege and Bertrand Russell, mathematical logic for example, or derivatives of it, like nonmonotonic reasoning and so on. It's interesting from a history of science perspective that even very recently, these approaches have been almost wiped out from the mainstream and have been largely replaced—in the field that calls itself AI now—by probabilistic and statistical models. My question is, what do you think explains that shift and is it a step in the right direction?
Chomsky: I heard Pat Winston give a talk about this years ago. One of the points he made was that AI and robotics got to the point where you could actually do things that were useful, so it turned to the practical applications and somewhat, maybe not abandoned, but put to the side, the more fundamental scientific questions, just caught up in the success of the technology and achieving specific goals.
So it shifted to engineering..."I have to say, myself, that I was very skeptical about the original work [in AI]. I thought it was first of all way too optimistic, it was assuming you could achieve things that required real understanding of systems that were barely understood, and you just can't get to that understanding by throwing a complicated machine at it."
Chomsky: It became ... well, which is understandable, but would of course direct people away from the original questions. I have to say, myself, that I was very skeptical about the original work. I thought it was first of all way too optimistic, it was assuming you could achieve things that required real understanding of systems that were barely understood, and you just can't get to that understanding by throwing a complicated machine at it. If you try to do that you are led to a conception of success, which is self-reinforcing, because you do get success in terms of this conception, but it's very different from what's done in the sciences. So for example, take an extreme case, suppose that somebody says he wants to eliminate the physics department and do it the right way. The "right" way is to take endless numbers of videotapes of what's happening outside the video, and feed them into the biggest and fastest computer, gigabytes of data, and do complex statistical analysis—you know, Bayesian this and that [Editor's note: A modern approach to analysis of data which makes heavy use of probability theory.]—and you'll get some kind of prediction about what's gonna happen outside the window next. In fact, you get a much better prediction than the physics department will ever give. Well, if success is defined as getting a fair approximation to a mass of chaotic unanalyzed data, then it's way better to do it this way than to do it the way the physicists do, you know, no thought experiments about frictionless planes and so on and so forth. But you won't get the kind of understanding that the sciences have always been aimed at—what you'll get at is an approximation to what's happening.
And that's done all over the place. Suppose you want to predict tomorrow's weather. One way to do it is okay I'll get my statistical priors, if you like, there's a high probability that tomorrow's weather here will be the same as it was yesterday in Cleveland, so I'll stick that in, and where the sun is will have some effect, so I'll stick that in, and you get a bunch of assumptions like that, you run the experiment, you look at it over and over again, you correct it by Bayesian methods, you get better priors. You get a pretty good approximation of what tomorrow's weather is going to be. That's not what meteorologists do—they want to understand how it's working. And these are just two different concepts of what success means, of what achievement is. In my own field, language fields, it's all over the place. Like computational cognitive science applied to language, the concept of success that's used is virtually always this. So if you get more and more data, and better and better statistics, you can get a better and better approximation to some immense corpus of text, like everything in The Wall Street Journal archives—but you learn nothing about the language.
A very different approach, which I think is the right approach, is to try to see if you can understand what the fundamental principles are that deal with the core properties, and recognize that in the actual usage, there's going to be a thousand other variables intervening—kind of like what's happening outside the window, and you'll sort of tack those on later on if you want better approximations, that's a different approach. These are just two different concepts of science. The second one is what science has been since Galileo, that's modern science. The approximating unanalyzed data kind is sort of a new approach, not totally, there's things like it in the past. It's basically a new approach that has been accelerated by the existence of massive memories, very rapid processing, which enables you to do things like this that you couldn't have done by hand. But I think, myself, that it is leading subjects like computational cognitive science into a direction of maybe some practical applicability ..
… in engineering?
Chomsky: … But away from understanding. Yeah, maybe some effective engineering. And it's kind of interesting to see what happened to engineering. So like when I got to MIT, it was 1950s, this was an engineering school. There was a very good math department, physics department, but they were service departments. They were teaching the engineers tricks they could use. The electrical engineering department, you learned how to build a circuit. Well if you went to MIT in the 1960s, or now, it's completely different. No matter what engineering field you're in, you learn the same basic science and mathematics. And then maybe you learn a little bit about how to apply it. But that's a very different approach. And it resulted maybe from the fact that really for the first time in history, the basic sciences, like physics, had something really to tell engineers. And besides, technologies began to change very fast, so not very much point in learning the technologies of today if it's going to be different 10 years from now. So you have to learn the fundamental science that's going to be applicable to whatever comes along next. And the same thing pretty much happened in medicine. So in the past century, again for the first time, biology had something serious to tell to the practice of medicine, so you had to understand biology if you want to be a doctor, and technologies again will change. Well, I think that's the kind of transition from something like an art, that you learn how to practice -- an analog would be trying to match some data that you don't understand, in some fashion, maybe building something that will work—to science, what happened in the modern period, roughly Galilean science.
I see. Returning to the point about Bayesian statistics in models of language and cognition. You've argued famously that speaking of the probability of a sentence is unintelligible on its own ...
Chomsky: … Well you can get a number if you want, but it doesn't mean anything.
It doesn't mean anything. But it seems like there's almost a trivial way to unify the probabilistic method with acknowledging that there are very rich internal mental representations, comprised of rules and other symbolic structures, and the goal of probability theory is just to link noisy sparse data in the world with these internal symbolic structures. And that doesn't commit you to saying anything about how these structures were acquired—they could have been there all along, or there partially with some parameters being tuned, whatever your conception is. But probability theory just serves as a kind of glue between noisy data and very rich mental representations.
Chomsky: Well ... there's nothing wrong with probability theory, there's nothing wrong with statistics.
But does it have a role?
Chomsky: If you can use it, fine. But the question is what are you using it for? First of all, first question is, is there any point in understanding noisy data? Is there some point to understanding what's going on outside the window?
Well, we are bombarded with it [noisy data], it's one of Marr's examples, we are faced with noisy data all the time, from our retina to ...
Chomsky: That's true. But what he says is: Let's ask ourselves how the biological system is picking out of that noise things that are significant. The retina is not trying to duplicate the noise that comes in. It's saying I'm going to look for this, that and the other thing. And it's the same with say, language acquisition. The newborn infant is confronted with massive noise, what William James called "a blooming, buzzing confusion," just a mess. If say, an ape or a kitten or a bird or whatever is presented with that noise, that's where it ends. However, the human infants, somehow, instantaneously and reflexively, picks out of the noise some scattered subpart which is language-related. That's the first step. Well, how is it doing that? It's not doing it by statistical analysis, because the ape can do roughly the same probabilistic analysis. It's looking for particular things. So psycholinguists, neurolinguists, and others are trying to discover the particular parts of the computational system and of the neurophysiology that are somehow tuned to particular aspects of the environment. Well, it turns out that there actually are neural circuits which are reacting to particular kinds of rhythm, which happen to show up in language, like syllable length and so on. And there's some evidence that that's one of the first things that the infant brain is seeking—rhythmic structures. And going back to Gallistel and Marr, its got some computational system inside which is saying "okay, here's what I do with these things" and say, by nine months, the typical infant has rejected—eliminated from its repertoire—the phonetic distinctions that aren't used in its own language. So initially of course, any infant is tuned to any language. But say, a Japanese kid at nine months won't react to the R-L distinction anymore, that's kind of weeded out. So the system seems to sort out lots of possibilities and restrict it to just ones that are part of the language, and there's a narrow set of those. You can make up a non-language in which the infant could never do it, and then you're looking for other things. For example, to get into a more abstract kind of language, there's substantial evidence by now that such a simple thing as linear order, what precedes what, doesn't enter into the syntactic and semantic computational systems, they're just not designed to look for linear order. So you find overwhelmingly that more abstract notions of distance are computed and not linear distance, and you can find some neurophysiological evidence for this, too. Like if artificial languages are invented and taught to people, which use linear order, like you negate a sentence by doing something to the third word. People can solve the puzzle, but apparently the standard language areas of the brain are not activated—other areas are activated, so they're treating it as a puzzle not as a language problem. You need more work, but ...
You take that as convincing evidence that activation or lack of activation for the brain area ...
Chomsky: … It's evidence, you'd want more of course. But this is the kind of evidence, both on the linguistics side you look at how languages work—they don't use things like third word in sentence. Take a simple sentence like "Instinctively, Eagles that fly swim," well, "instinctively" goes with swim, it doesn't go with fly, even though it doesn't make sense. And that's reflexive. "Instinctively," the adverb, isn't looking for the nearest verb, it's looking for the structurally most prominent one. That's a much harder computation. But that's the only computation which is ever used. Linear order is a very easy computation, but it's never used. There's a ton of evidence like this, and a little neurolinguistic evidence, but they point in the same direction. And as you go to more complex structures, that's where you find more and more of that.
That's, in my view at least, the way to try to discover how the system is actually working, just like in vision, in Marr's lab, people like Shimon Ullman discovered some pretty remarkable things like the rigidity principle. You're not going to find that by statistical analysis of data. But he did find it by carefully designed experiments. Then you look for the neurophysiology, and see if you can find something there that carries out these computations. I think it's the same in language, the same in studying our arithmetical capacity, planning, almost anything you look at. Just trying to deal with the unanalyzed chaotic data is unlikely to get you anywhere, just like as it wouldn't have gotten Galileo anywhere. In fact, if you go back to this, in the 17th century, it wasn't easy for people like Galileo and other major scientists to convince the NSF [National Science Foundation] of the day—namely, the aristocrats—that any of this made any sense. I mean, why study balls rolling down frictionless planes, which don't exist. Why not study the growth of flowers? Well, if you tried to study the growth of flowers at that time, you would get maybe a statistical analysis of what things looked like."It's worth remembering that with regard to cognitive science, we're kind of pre-Galilean, just beginning to open up the subject."
It's worth remembering that with regard to cognitive science, we're kind of pre-Galilean, just beginning to open up the subject. And I think you can learn something from the way science worked [back then]. In fact, one of the founding experiments in history of chemistry, was about 1640 or so, when somebody proved to the satisfaction of the scientific world, all the way up to Newton, that water can be turned into living matter. The way they did it was—of course, nobody knew anything about photosynthesis—so what you do is you take a pile of earth, you heat it so all the water escapes. You weigh it, and put it in a branch of a willow tree, and pour water on it, and measure you the amount of water you put in. When you're done, you the willow tree is grown, you again take the earth and heat it so all the water is gone—same as before. Therefore, you've shown that water can turn into an oak tree or something. It is an experiment, it's sort of right, but it's just that you don't know what things you ought to be looking for. And they weren't known until Priestly found that air is a component of the world, it's got nitrogen, and so on, and you learn about photosynthesis and so on. Then you can redo the experiment and find out what's going on. But you can easily be misled by experiments that seem to work because you don't know enough about what to look for. And you can be misled even more if you try to study the growth of trees by just taking a lot of data about how trees growing, feeding it into a massive computer, doing some statistics and getting an approximation of what happened.
In the domain of biology, would you consider the work of Mendel, as a successful case, where you take this noisy data— essentially counts—and you leap to postulate this theoretical object ...
Chomsky: … Well, throwing out a lot of the data that didn't work.
… But seeing the ratio that made sense, given the theory.
Chomsky: Yeah, he did the right thing. He let the theory guide the data. There was counter data which was more or less dismissed, you know you don't put it in your papers. And he was of course talking about things that nobody could find, like you couldn't find the units that he was postulating. But that's, sure, that's the way science works. Same with chemistry. Chemistry, until my childhood, not that long ago, was regarded as a calculating device. Because you couldn't reduce to physics. So it's just some way of calculating the result of experiments. The Bohr atom was treated that way. It's the way of calculating the results of experiments but it can't be real science, because you can't reduce it to physics, which incidentally turned out to be true, you couldn't reduce it to physics because physics was wrong. When quantum physics came along, you could unify it with virtually unchanged chemistry. So the project of reduction was just the wrong project. The right project was to see how these two ways of looking at the world could be unified. And it turned out to be a surprise—they were unified by radically changing the underlying science. That could very well be the case with say, psychology and neuroscience. I mean, neuroscience is nowhere near as advanced as physics was a century ago.
That would go against the reductionist approach of looking for molecules that are correlates of ...
Chomsky: Yeah. In fact, the reductionist approach has often been shown to be wrong. The unification approach makes sense. But unification might not turn out to be reduction, because the core science might be misconceived as in the physics-chemistry case and I suspect very likely in the neuroscience-psychology case. If Gallistel is right, that would be a case in point that yeah, they can be unified, but with a different approach to the neurosciences.
So is that a worthy goal of unification or the fields should proceed in parallel?
Chomsky: Well, unification is kind of an intuitive ideal, part of the scientific mystique, if you like. It's that you're trying to find a unified theory of the world. Now maybe there isn't one, maybe different parts work in different ways, but your assumption is until I'm proven wrong definitively, I'll assume that there's a unified account of the world, and it's my task to try to find it. And the unification may not come out by reduction—it often doesn't. And that's kind of the guiding logic of David Marr's approach: what you discover at the computational level ought to be unified with what you'll some day find out at the mechanism level, but maybe not in terms of the way we now understand the mechanisms.
And implicit in Marr it seems that you can't work on all three in parallel [computational, algorithmic, implementation levels], it has to proceed top-down, which is a very stringent requirement, given that science usually doesn't work that way.
Chomsky: Well, he wouldn't have said it has to be rigid. Like for example, discovering more about the mechanisms might lead you to change your concept of computation. But there's kind of a logical precedence, which isn't necessarily the research precedence, since in research everything goes on at the same time. But I think that the rough picture is okay. Though I should mention that Marr's conception was designed for input systems ...
information-processing systems ...
Chomsky: Yeah, like vision. There's some data out there—it's a processing system —and something goes on inside. It isn't very well designed for cognitive systems. Like take your capacity to take out arithmetical operations ...
It's very poor, but yeah ...
Chomsky: Okay [laughs]. But it's an internal capacity, you know your brain is a controlling unit of some kind of Turing machine, and it has access to some external data, like memory, time and so on. And in principle, you could multiply anything, but of course not in practice. If you try to find out what that internal system is of yours, the Marr hierarchy doesn't really work very well. You can talk about the computational level—maybe the rules I have are Peano's axioms [Editor's note: a mathematical theory (named after Italian mathematician Giuseppe Peano) that describes a core set of basic rules of arithmetic and natural numbers, from which many useful facts about arithmetic can be deduced], or something, whatever they are—that's the computational level. In theory, though we don't know how, you can talk about the neurophysiological level, nobody knows how, but there's no real algorithmic level. Because there's no calculation of knowledge, it's just a system of knowledge. To find out the nature of the system of knowledge, there is no algorithm, because there is no process. Using the system of knowledge, that'll have a process, but that's something different.
But since we make mistakes, isn't that evidence of a process gone wrong?
Chomsky: That's the process of using the internal system. But the internal system itself is not a process, because it doesn't have an algorithm. Take, say, ordinary mathematics. If you take Peano's axioms and rules of inference, they determine all arithmetical computations, but there's no algorithm. If you ask how does a number theoretician applies these, well all kinds of ways. Maybe you don't start with the axioms and start with the rules of inference. You take the theorem, and see if I can establish a lemma, and if it works, then see if I can try to ground this lemma in something, and finally you get a proof which is a geometrical object.
But that's a fundamentally different activity from me adding up small numbers in my head, which surely does have some kind of algorithm.
Chomsky: Not necessarily. There's an algorithm for the process in both cases. But there's no algorithm for the system itself, it's kind of a category mistake. You don't ask the question what's the process defined by Peano's axioms and the rules of inference, there's no process. There can be a process of using them. And it could be a complicated process, and the same is true of your calculating. The internal system that you have—for that, the question of process doesn't arise. But for your using that internal system, it arises, and you may carry out multiplications all kinds of ways. Like maybe when you add 7 and 6, let's say, one algorithm is to say "I'll see how much it takes to get to 10"—it takes 3, and now I've got 4 left, so I gotta go from 10 and add 4, I get 14. That's an algorithm for adding -- it's actually one I was taught in kindergarten. That's one way to add.
But there are other ways to add—there's no kind of right algorithm. These are algorithms for carrying out the process the cognitive system that's in your head. And for that system, you don't ask about algorithms. You can ask about the computational level, you can ask about the mechanism level. But the algorithm level doesn't exist for that system. It's the same with language. Language is kind of like the arithmetical capacity. There's some system in there that determines the sound and meaning of an infinite array of possible sentences. But there's no question about what the algorithm is. Like there's no question about what a formal system of arithmetic tells you about proving theorems. The use of the system is a process and you can study it in terms of Marr's level. But it's important to be conceptually clear about these distinctions.
It just seems like an astounding task to go from a computational level theory, like Peano axioms, to Marr level 3 of the ...
Chomsky: mechanisms ...
… mechanisms and implementations ...
Chomsky: Oh yeah. Well ...
… without an algorithm at least.
Chomsky: Well, I don't think that's true. Maybe information about how it's used, that'll tell you something about the mechanisms. But some higher intelligence—maybe higher than ours—would see that there's an internal system, its got a physiological basis, and I can study the physiological basis of that internal system. Not even looking at the process by which it's used. Maybe looking at the process by which it's used maybe gives you helpful information about how to proceed. But it's conceptually a different problem. That's the question of what's the best way to study something. So maybe the best way to study the relation between Peano's axioms and neurons is by watching mathematicians prove theorems. But that's just because it'll give you information that may be helpful. The actual end result of that will be an account of the system in the brain, the physiological basis for it, with no reference to any algorithm. The algorithms are about a process of using it, which may help you get answers. Maybe like incline planes tell you something about the rate of fall, but if you take a look at Newton's laws, they don't say anything about incline planes.
Right. So the logic for studying cognitive and language systems using this kind of Marr approach makes sense, but since you've argued that language capacity is part of the genetic endowment, you could apply it to other biological systems, like the immune system, the circulatory system ...
Chomsky: Certainly, I think it's very similar. You can say the same thing about study of the immune system.
It might even be simpler, in fact, to do it for those systems than for cognition.
Chomsky: Though you'd expect different answers. You can do it for the digestive system. Suppose somebody's studying the digestive system. Well, they're not going to study what happens when you have a stomach flu, or when you've just eaten a big Mac, or something. Let's go back to taking pictures outside the window. One way of studying the digestive system is just to take all data you can find about what digestive systems do under any circumstances, toss the data into a computer, do statistical analysis -- you get something. But it's not gonna be what any biologist would do. They want to abstract away, at the very beginning, from what are presumed— maybe wrongly, you can always be wrong—irrelevant variables, like do you have stomach flu.
But that's precisely what the biologists are doing, they are taking the sick people with the sick digestive system, comparing them to the normals, and measuring these molecular properties.
Chomsky: They're doing it in an advanced stage. They already understand a lot about the study of the digestive system before we compare them, otherwise you wouldn't know what to compare, and why is one sick and one isn't.
Well, they're relying on statistical analysis to pick out the features that discriminate. It's a highly fundable approach, because you're claiming to study sick people.
Chomsky: It may be the way to fund things. Like maybe the way to fund study of language is to say, maybe help cure autism. That's a different question [laughs]. But the logic of the search is to begin by studying the system abstracted from what you, plausibly, take to be irrelevant intrusions, see if you can find its basic nature—then ask, well, what happens when I bring in some of this other stuff, like stomach flu.
It still seems like there's a difficulty in applying Marr's levels to these kinds of systems. If you ask, what is the computational problem that the brain is solving, we have kind of an answer, it's sort of like a computer. But if you ask, what is the computational problem that's being solved by the lung, that's very difficult to even think— it's not obviously an information-processing kind of problem."There's no reason to assume that all of biology is computational. There may be reasons to assume that cognition is."
Chomsky: No, but there's no reason to assume that all of biology is computational. There may be reasons to assume that cognition is. And in fact Gallistel is not saying that everything is in the body ought to be studied by finding read/write/address units.
It just seems contrary to any evolutionary intuition. These systems evolved together, reusing many of the same parts, same molecules, pathways. Cells are computing things.
Chomsky: You don't study the lung by asking what cells compute. You study the immune system and the visual system, but you're not going to expect to find the same answers. An organism is a highly modular system, has a lot of complex subsystems, which are more or less internally integrated. They operate by different principles. The biology is highly modular. You don't assume it's all just one big mess, all acting the same way.
No, sure, but I'm saying you would apply the same approach to study each of the modules.
Chomsky: Not necessarily, not if the modules are different. Some of the modules may be computational, others may not be.
So what would you think would be an adequate theory that is explanatory, rather than just predicting data, the statistical way, what would be an adequate theory of these systems that are not computing systems—can we even understand them?
Chomsky: Sure. You can understand a lot about say, what makes an embryo turn into a chicken rather than a mouse, let's say. It's a very intricate system, involves all kinds of chemical interactions, all sorts of other things. Even the nematode, it's by no means obviously—in fact there are reports from the study here—that it's all just a matter of a neural net. You have to look into complex chemical interactions that take place in the brain, in the nervous system. You have to look into each system on its own. These chemical interactions might not be related to how your arithmetical capacity works —probably aren't. But they might very well be related to whether you decide to raise your arm or lower it.
Though if you study the chemical interactions it might lead you into what you've called just a redescription of the phenomena.
Chomsky: Or an explanation. Because maybe that's directly, crucially, involved.
But if you explain it in terms of chemical X has to be turned on, or gene X has to be turned on, you've not really explained how organism-determination is done. You've simply found a switch, and hit that switch.
Chomsky: But then you look further, and find out what makes this gene do such and such under these circumstances, and do something else under different circumstances.
But if genes are the wrong level of abstraction, you'd be screwed.
Chomsky: Then you won't get the right answer. And maybe they're not. For example, it's notoriously difficult to account for how an organism arises from a genome. There's all kinds of production going on in the cell. If you just look at gene action, you may not be in the right level of abstraction. You never know, that's what you try to study. I don't think there's any algorithm for answering those questions, you try.
So I want to shift gears more toward evolution. You've criticized a very interesting position you've called "phylogenetic empiricism." You've criticized this position for not having explanatory power. It simply states that: well, the mind is the way it because of adaptations to the environment that were selected for. And these were selected for by natural selection. You've argued that this doesn't explain anything because you can always appeal to these two principles of mutation and selection.
Chomsky: Well you can wave your hands at them, but they might be right. It could be that, say, the development of your arithmetical capacity, arose from random mutation and selection. If it turned out to be true, fine.
It seems like a truism.
Chomsky: Well, I mean, doesn't mean it's false. Truisms are true. [laughs].
But they don't explain much."Why do cells split into spheres and not cubes? It's not random mutation and natural selection; it's a law of physics."
Chomsky: Maybe that's the highest level of explanation you can get. You can invent a world—I don't think it's our world—but you can invent a world in which nothing happens except random changes in objects and selection on the basis of external forces. I don't think that's the way our world works, I don't think it's the way any biologist thinks it is. There are all kind of ways in which natural law imposes channels within which selection can take place, and some things can happen and other things don't happen. Plenty of things that go on in the biology in organisms aren't like this. So take the first step, meiosis. Why do cells split into spheres and not cubes? It's not random mutation and natural selection; it's a law of physics. There's no reason to think that laws of physics stop there, they work all the way through.
Well, they constrain the biology, sure.
Chomsky: Okay, well then it's not just random mutation and selection. It's random mutation, selection, and everything that matters, like laws of physics.
So is there room for these approaches which are now labeled "comparative genomics," like the Broad Institute here [at MIT/Harvard] is generating massive amounts of data, of different genomes, different animals, different cells under different conditions and sequencing any molecule that is sequenceable. Is there anything that can be gleaned about these high-level cognitive tasks from these comparative evolutionary studies or is it premature?
Chomsky: I am not saying it's the wrong approach, but I don't know anything that can be drawn from it. Nor would you expect to.
You don't have any examples where this evolutionary analysis has informed something? Like Foxp2 mutations? [Editor's note: A gene that is thought be implicated in speech or language capacities. A family with a stereotyped speech disorder was found to have genetic mutations that disrupt this gene. This gene evolved to have several mutations unique to the human evolutionary lineage.]
Chomsky: Foxp2 is kind of interesting, but it doesn't have anything to do with language. It has to do with fine motor coordinations and things like that. Which takes place in the use of language, like when you speak you control your lips and so on, but all that's very peripheral to language, and we know that. So for example, whether you use the articulatory organs or sign, you know hand motions, it's the same language. In fact, it's even being analyzed and produced in the same parts of the brain, even though one of them is moving your hands and the other is moving your lips. So whatever the externalization is, it seems quite peripheral. I think they're too complicated to talk about, but I think if you look closely at the design features of language, you get evidence for that. There are interesting cases in the study of language where you find conflicts between computational efficiency and communicative efficiency.
Take this case I even mentioned of linear order. If you want to know which verb the adverb attaches to, the infant reflexively using minimal structural distance, not minimal linear distance. Well, it's using minimal linear distances, computationally easy, but it requires having linear order available. And if linear order is only a reflex of the sensory-motor system, which makes sense, it won't be available. That's evidence that the mapping of the internal system to the sensory-motor system is peripheral to the workings of the computational system.
But it might constrain it like physics constrains meiosis?
Chomsky: It might, but there's very little evidence of that. So for example the left end—left in the sense of early—of a sentence has different properties from the right end. If you want to ask a question, let's say "Who did you see?" You put the "Who" in front, not in the end. In fact, in every language in which a wh-phrase -- like who, or which book, or something—moves to somewhere else, it moves to the left, not to the right. That's very likely a processing constraint. The sentence opens by telling you, the hearer, here's what kind of a sentence it is. If it's at the end, you have to have the whole declarative sentence, and at the end you get the information I'm asking about. If you spell it out, it could be a processing constraint. So that's a case, if true, in which the processing constraint, externalization, do affect the computational character of the syntax and semantics.
There are cases where you find clear conflicts between computational efficiency and communicative efficiency. Take a simple case, structural ambiguity. If I say, "Visiting relatives can be a nuisance"—that's ambiguous. Relatives that visit, or going to visit relatives. It turns out in every such case that's known, the ambiguity is derived by simply allowing the rules to function freely, with no constraints, and that sometimes yields ambiguities. So it's computationally efficient, but it's inefficient for communication, because it leads to unresolvable ambiguity.
And in fact, every case of a conflict that's known, computational efficiency wins. The externalization is yielding all kinds of ambiguities but for simple computational reasons, it seems that the system internally is just computing efficiently, it doesn't care about the externalization. Well, I haven't made that a very plausible, but if you spell it out it can be made quite a convincing argument I think.
That tells something about evolution. What it strongly suggests is that in the evolution of language, a computational system developed, and later on it was externalized. And if you think about how a language might have evolved, you're almost driven to that position. At some point in human evolution, and it's apparently pretty recent given the archeological record—maybe last hundred thousand years, which is nothing—at some point a computational system emerged with had new properties, that other organisms don't have, that has kind of arithmetical type properties ...
It enabled better thought before externalization?
Chomsky: It gives you thought. Some rewiring of the brain, that happens in a single person, not in a group. So that person had the capacity for thought—the group didn't. So there isn't any point in externalization. Later on, if this genetic change proliferates, maybe a lot of people have it, okay then there's a point in figuring out a way to map it to the sensory-motor system and that's externalization but it's a secondary process.
Unless the externalization and the internal thought system are coupled in ways we just don't predict.
Chomsky: We don't predict, and they don't make a lot of sense. Why should it be connected to the external system? In fact, say your arithmetical capacity isn't. And there are other animals, like songbirds, which have internal computational systems, bird song. It's not the same system but it's some kind of internal computational system. And it is externalized, but sometimes it's not. A chick in some species acquires the song of that species but doesn't produce it until maturity. During that early period it has the song, but it doesn't have the externalization system. Actually that's true of humans too, like a human infant understands a lot more than it can produce—plenty of experimental evidence for this, meaning it's got the internal system somehow, but it can't externalize it. Maybe it doesn't have enough memory, or whatever it may be.
Chomsky: Philosophy of science is a very interesting field, but I don't think it really contribute to science, it learns from science. It tries to understand what the sciences do, why do they achieve things, what are the wrong paths, see if we can codify that and come to understand. What I think is valuable is the history of science. I think we learn a lot of things from the history of science that can be very valuable to the emerging sciences. Particularly when we realize that in say, the emerging cognitive sciences, we really are in a kind of pre-Galilean stage. We don't know what we're looking for anymore than Galileo did, and there's a lot to learn from that. So for example one striking fact about early science, not just Galileo, but the Galilean breakthrough, was the recognition that simple things are puzzling.
Take say, if I'm holding this here [cup of water], and say the water is boiling [putting hand over water], the steam will rise, but if I take my hand away the cup will fall. Well why does the cup fall and the steam rise? Well for millennia there was a satisfactory answer to that: they're seeking their natural place.
Like in Aristotelian physics?
Chomsky: That's the Aristotelian physics. The best and greatest scientists thought that was answer. Galileo allowed himself to be puzzled by it. As soon as you allow yourself to be puzzled by it, you immediately find that all your intuitions are wrong. Like the fall of a big mass and a small mass, and so on. All your intuitions are wrong—there are puzzles everywhere you look. That's something to learn from the history of science. Take the one example that I gave to you, "Instinctively eagles that fly swim." Nobody ever thought that was puzzling—yeah, why not. But if you think about it, it's very puzzling, you're using a complex computation instead of a simple one. Well, if you allow yourself to be puzzled by that, like the fall of a cup, you ask "Why?" and then you're led down a path to some pretty interesting answers. Like maybe linear order just isn't part of the computational system, which is a strong claim about the architecture of the mind —it says it's just part of the externalization system, secondary, you know. And that opens up all sorts of other paths, same with everything else.
Take another case: the difference between reduction and unification. History of science gives some very interesting illustrations of that, like chemistry and physics, and I think they're quite relevant to the state of the cognitive and neurosciences today.
[2] Norvig's rebuttal. Do go through the comments section of this link
On Chomsky and the Two Cultures of Statistical Learninghttp://norvig.com/chomsky.html
On Chomsky and the Two Cultures of Statistical Learning
At the Brains, Minds, and Machines symposium held during MIT's 150th birthday party, Technology Review reports that Prof. Noam Chomskyderided researchers in machine learning who use purely statistical methods to produce behavior that mimics something in the world, but who don't try to understand the meaning of that behavior.The transcript is now available, so let's quote Chomsky himself:
It's true there's been a lot of work on trying to apply statistical models to various linguistic problems. I think there have been some successes, but a lot of failures. There is a notion of success ... which I think is novel in the history of science. It interprets success as approximating unanalyzed data.
This essay discusses what Chomsky said, speculates on what he might have meant, and tries to determine the truth and importance of his claims.
Chomsky's remarks were in response to Steven Pinker's question about the success of probabilistic models trained with statistical methods.
- What did Chomsky mean, and is he right?
- What is a statistical model?
- How successful are statistical language models?
- Is there anything like their notion of success in the history of science?
- What doesn't Chomsky like about statistical models?
What did Chomsky mean, and is he right?
I take Chomsky's points to be the following:- Statistical language models have had engineering success, but that is irrelevant to science.
- Accurately modeling linguistic facts is just butterfly collecting; what matters in science (and specifically linguistics) is the underlying principles.
- Statistical models are incomprehensible; they provide no insight.
- Statistical models may provide an accurate simulation of some phenomena, but the simulation is done completely the wrong way; people don't decide what the third word of a sentence should be by consulting a probability table keyed on the previous two words, rather they map from an internal semantic form to a syntactic tree-structure, which is then linearized into words. This is done without any probability or statistics.
- Statistical models have been proven incapable of learning language; therefore language must be innate, so why are these statistical modelers wasting their time on the wrong enterprise?
- I agree that engineering success is not the goal or the measure of science. But I observe that science and engineering develop together, and that engineering success shows that something is working right, and so is evidence (but not proof) of a scientifically successful model.
- Science is a combination of gathering facts and making theories; neither can progress on its own. I think Chomsky is wrong to push the needle so far towards theory over facts; in the history of science, the laborious accumulation of facts is the dominant mode, not a novelty. The science of understanding language is no different than other sciences in this respect.
- I agree that it can be difficult to make sense of a model containing billions of parameters. Certainly a human can't understand such a model by inspecting the values of each parameter individually. But one can gain insight by examing the properties of the model—where it succeeds and fails, how well it learns as a function of data, etc.
- I agree that a Markov model of word probabilities cannot model all of language. It is equally true that a concise tree-structure model without probabilities cannot model all of language. What is needed is a probabilistic model that covers words, trees, semantics, context, discourse, etc. Chomsky dismisses all probabilistic models because of shortcomings of particular 50-year old models. I understand how Chomsky arrives at the conclusion that probabilistic models are unnecessary, from his study of the generation of language. But the vast majority of people who study interpretation tasks, such as speech recognition, quickly see that interpretation is an inherently probabilistic problem: given a stream of noisy input to my ears, what did the speaker most likely mean? Einstein said to make everything as simple as possible, but no simpler. Many phenomena in science are stochastic, and the simplest model of them is a probabilistic model; I believe language is such a phenomenon and therefore that probabilistic models are our best tool for representing facts about language, for algorithmically processing language, and for understanding how humans process language.
- In 1967, Gold's Theorem showed some theoretical limitations of logical deduction on formal mathematical languages. But this result has nothing to do with the task faced by learners of natural language. In any event, by 1969 we knew that probabilistic inference (over probabilistic context-free grammars) is not subject to those limitations (Horning showed that learning of PCFGs is possible). I agree with Chomsky that it is undeniable that humans have some innate capability to learn natural language, but we don't know enough about that capability to rule out probabilistic language representations, nor statistical learning. I think it is much more likely that human language learning involves something like probabilistic and statistical inference, but we just don't know yet.
What is a statistical model?
A statistical model is a mathematical model which is modified or trained by the input of data points. Statistical models are often but not always probabilistic. Where the distinction is important we will be careful not to just say "statistical" but to use the following component terms:- A mathematical model specifies a relation among variables, either in functional form that maps inputs to outputs (e.g. y = m x + b) or in relation form (e.g. the following (x, y) pairs are part of the relation).
- A probabilistic model specifies a probability distribution over possible values of random variables, e.g., P(x, y), rather than a strict deterministic relationship, e.g., y = f(x).
- A trained model uses some training/learning algorithm to take as input a collection of possible models and a collection of data points (e.g. (x, y) pairs) and select the best model. Often this is in the form of choosing the values of parameters (such as m and b above) through a process of statistical inference.
For example, a decade before Chomsky, Claude Shannon proposed probabilistic models of communication based on Markov chains of words. If you have a vocabulary of 100,000 words and a second-order Markov model in which the probability of a word depends on the previous two words, then you need a quadrillion (1015) probability values to specify the model. The only feasible way to learn these 1015 values is to gather statistics from data and introduce some smoothing method for the many cases where there is no data. Therefore, most (but not all) probabilistic models are trained. Also, many (but not all) trained models are probabilistic.
As another example, consider the Newtonian model of gravitational attraction, which says that the force between two objects of mass m1 and m2 a distance r apart is given by
F = G m1 m2 / r2where G is the universal gravitational constant. This is a trained model because the gravitational constant G is determined by statistical inference over the results of a series of experiments that contain stochastic experimental error. It is also a deterministic (non-probabilistic) model because it states an exact functional relationship. I believe that Chomsky has no objection to this kind of statistical model. Rather, he seems to reserve his criticism for statistical models like Shannon's that have quadrillions of parameters, not just one or two.
(This example brings up another distinction: the gravitational model is continuous and quantitative whereas the linguistic tradition has favored models that are discrete, categorical, and qualitative: a word is or is not a verb, there is no question of its degree of verbiness. For more on these distinctions, see Chris Manning's article on Probabilistic Syntax.)
A relevant probabilistic statistical model is the ideal gas law, which describes the pressure P of a gas in terms of the the number of molecules, N, the temperature T, and Boltzmann's constant, K:
P = N k T / V.
The equation can be derived from first principles using the tools of statistical mechanics. It is an uncertain, incorrect model; the true model would have to describe the motions of individual gas molecules. This model ignores that complexity and summarizes our uncertainty about the location of individual molecules. Thus, even though it is statistical and probabilistic, even though it does not completely model reality, it does provide both good predictions and insight—insight that is not available from trying to understand the true movements of individual molecules.
Now let's consider the non-statistical model of spelling expressed by the rule "I before E except after C." Compare that to the probabilistic, trained statistical model:
This model comes from statistics on a corpus of a trillion words of English text. The notation P(IE) is the probability that a word sampled from this corpus contains the consecutive letters "IE." P(CIE) is the probability that a word contains the consecutive letters "CIE", and P(*IE) is the probability of any letter other than C followed by IE. The statistical data confirms that IE is in fact more common than EI, and that the dominance of IE lessens wehn following a C, but contrary to the rule, CIE is still more common than CEI. Examples of "CIE" words include "science," "society," "ancient" and "species." The disadvantage of the "I before E except after C" model is that it is not very accurate. Consider:P(IE) = 0.0177 P(CIE) = 0.0014 P(*IE) = 0.163 P(EI) = 0.0046 P(CEI) = 0.0005 P(*EI) = 0.0041
Accuracy("I before E") = 0.0177/(0.0177+0.0046) = 0.793 Accuracy("I before E except after C") = (0.0005+0.0163)/(0.0005+0.0163+0.0014+0.0041) = 0.753A more complex statistical model (say, one that gave the probability of all 4-letter sequences, and/or of all known words) could be ten times more accurate at the task of spelling, but offers little insight into what is going on. (Insight would require a model that knows about phonemes, syllabification, and language of origin. Such a model could be trained (or not) and probabilistic (or not).)
As a final example (not of statistical models, but of insight), consider the Theory of Supreme Court Justice Hand-Shaking: when the supreme court convenes, all attending justices shake hands with every other justice. The number of attendees, n, must be an integer in the range 0 to 9; what is the total number of handshakes, h for a given n? Here are three possible explanations:
- Each of n justices shakes hands with the other n - 1 justices, but that counts Alito/Breyer and Breyer/Alito as two separate shakes, so we should cut the total in half, and we end up with h = n × (n - 1) / 2.
- To avoid double-counting, we will order the justices by seniority and only count a more-senior/more-junior handshake, not a more-junior/more-senior one. So we count, for each justice, the shakes with the more junior justices, and sum them up, giving h = Σi = 1 .. n (i - 1).
- Just look at this table:
n: 0 1 2 3 4 5 6 7 8 9 h: 0 0 1 3 6 10 15 21 28 36
How successful are statistical language models?
Chomsky said words to the effect that statistical language models have had some limited success in some application areas. Let's look at computer systems that deal with language, and at the notion of "success" defined by "making accurate predictions about the world." First, the major application areas:- Search engines: 100% of major players are trained and probabilistic. Their operation cannot be described by a simple function.
- Speech recognition: 100% of major systems are trained and probabilistic, mostly relying on probabilistic hidden Markov models.
- Machine translation: 100% of top competitors in competitions such as NIST use statistical methods. Some commercial systems use a hybrid of trained and rule-based approaches. Of the 4000 language pairs covered by machine translation systems, a statistical system is by far the best for every pair except Japanese-English, where the top statistical system is roughly equal to the top hybrid system.
- Question answering: this application is less well-developed, and many systems build heavily on the statistical and probabilistic approach used by search engines. The IBM Watson system that recently won on Jeopardy is thoroughly probabilistic and trained, while Boris Katz's START is a hybrid. All systems use at least some statistical techniques.
Now let's look at some components that are of interest only to the computational linguist, not to the end user:
- Word sense disambiguation: 100% of top competitors at the SemEval-2 competition used statistical techniques; most are probabilistic; some use a hybrid approach incorporating rules from sources such as Wordnet.
- Coreference resolution: The majority of current systems are statistical, although we should mention the system of Haghighi and Klein, which can be described as a hybrid system that is mostly rule-based rather than trained, and performs on par with top statistical systems.
- Part of speech tagging: Most current systems are statistical. The Brill tagger stands out as a successful hybrid system: it learns a set of deterministic rules from statistical data.
- Parsing: There are many parsing systems, using multiple approaches. Almost all of the most successful are statistical, and the majority are probabilistic (with a substantial minority of deterministic parsers).
Clearly, it is inaccurate to say that statistical models (and probabilistic models) have achieved limited success; rather they have achieved a dominant (although not exclusive) position.
Another measure of success is the degree to which an idea captures a community of researchers. As Steve Abney wrote in 1996, "In the space of the last ten years, statistical methods have gone from being virtually unknown in computational linguistics to being a fundamental given. ... anyone who cannot at least use the terminology persuasively risks being mistaken for kitchen help at the ACL [Association for Computational Linguistics] banquet."
Now of course, the majority doesn't rule -- just because everyone is jumping on some bandwagon, that doesn't make it right. But I made the switch: after about 14 years of trying to get language models to work using logical rules, I started to adopt probabilistic approaches (thanks to pioneers like Gene Charniak (and Judea Pearl for probability in general) and to my colleagues who were early adopters, like Dekai Wu). And I saw everyone around me making the same switch. (And I didn't see anyone going in the other direction.) We all saw the limitations of the old tools, and the benefits of the new.
And while it may seem crass and anti-intellectual to consider a financial measure of success, it is worth noting that the intellectual offspring of Shannon's theory create several trillion dollars of revenue each year, while the offspring of Chomsky's theories generate well under a billion.
This section has shown that one reason why the vast majority of researchers in computational linguistics use statistical models is an engineering reason: statistical models have state-of-the-art performance, and in most cases non-statistical models perform worst. For the remainder of this essay we will concentrate on scientific reasons: that probabilistic models better represent linguistic facts, and statistical techniques make it easier for us to make sense of those facts.
Is there anything like [the statistical model] notion of success in the history of science?
When Chomsky said "That's a notion of [scientific] success that's very novel. I don't know of anything like it in the history of science" he apparently meant that the notion of success of "accurately modeling the world" is novel, and that the only true measure of success in the history of science is "providing insight" — of answering why things are the way they are, not just describing how they are.A dictionary definition of science is "the systematic study of the structure and behavior of the physical and natural world through observation and experiment," which stresses accurate modeling over insight, but it seems to me that both notions have always coexisted as part of doing science. To test that, I consulted the epitome of doing science, namely Science. I looked at the current issue and chose a title and abstract at random:
Chlorinated Indium Tin Oxide Electrodes with High Work Function for Organic Device CompatibilityIn organic light-emitting diodes (OLEDs), a stack of multiple organic layers facilitates charge flow from the low work function [~4.7 electron volts (eV)] of the transparent electrode (tin-doped indium oxide, ITO) to the deep energy levels (~6 eV) of the active light-emitting organic materials. We demonstrate a chlorinated ITO transparent electrode with a work function of >6.1 eV that provides a direct match to the energy levels of the active light-emitting materials in state-of-the art OLEDs. A highly simplified green OLED with a maximum external quantum efficiency (EQE) of 54% and power efficiency of 230 lumens per watt using outcoupling enhancement was demonstrated, as were EQE of 50% and power efficiency of 110 lumens per watt at 10,000 candelas per square meter.
It certainly seems that this article is much more focused on "accurately modeling the world" than on "providing insight." The paper does indeed fit in to a body of theories, but it is mostly reporting on specific experiments and the results obtained from them (e.g. efficiency of 54%).
I then looked at all the titles and abstracts from the current issue of Science:
- Comparative Functional Genomics of the Fission Yeasts
- Dimensionality Control of Electronic Phase Transitions in Nickel-Oxide Superlattices
- Competition of Superconducting Phenomena and Kondo Screening at the Nanoscale
- Chlorinated Indium Tin Oxide Electrodes with High Work Function for Organic Device Compatibility
- Probing Asthenospheric Density, Temperature, and Elastic Moduli Below the Western United States
- Impact of Polar Ozone Depletion on Subtropical Precipitation
- Fossil Evidence on Origin of the Mammalian Brain
- Industrial Melanism in British Peppered Moths Has a Singular and Recent Mutational Origin
- The Selaginella Genome Identifies Genetic Changes Associated with the Evolution of Vascular Plants
- Chromatin "Prepattern" and Histone Modifiers in a Fate Choice for Liver and Pancreas
- Spatial Coupling of mTOR and Autophagy Augments Secretory Phenotypes
- Diet Drives Convergence in Gut Microbiome Functions Across Mammalian Phylogeny and Within Humans
- The Toll-Like Receptor 2 Pathway Establishes Colonization by a Commensal of the Human Microbiota
- A Packing Mechanism for Nucleosome Organization Reconstituted Across a Eukaryotic Genome
- Structures of the Bacterial Ribosome in Classical and Hybrid States of tRNA Binding
- Mapping the NPHP-JBTS-MKS Protein Network Reveals Ciliopathy Disease Genes and Pathways
- Double-Strand Break Repair-Independent Role for BRCA2 in Blocking Stalled Replication Fork Degradation by MRE11
- Establishment and Maintenance of Alternative Chromatin States at a Multicopy Gene Locus
- An Epigenetic Signature for Monoallelic Olfactory Receptor Expression
- Distinct p53 Transcriptional Programs Dictate Acute DNA-Damage Responses and Tumor Suppression
- An ADIOL-ERβ-CtBP Transrepression Pathway Negatively Regulates Microglia-Mediated Inflammation
- A Hormone-Dependent Module Regulating Energy Balance
- Class IIa Histone Deacetylases Are Hormone-Activated Regulators of FOXO and Mammalian Glucose Homeostasis
- Physics: for groundbreaking experiments regarding the two-dimensional material graphene
- Chemistry: for palladium-catalyzed cross couplings in organic synthesis
- Physiology or Medicine: for the development of in vitro fertilization
What doesn't Chomsky like about statistical models?
I said that statistical models are sometimes confused with probabilistic models; let's first consider the extent to which Chomsky's objections are actually about probabilistic models. In 1969 he famously wrote:
But it must be recognized that the notion of "probability of a sentence" is an entirely useless one, under any known interpretation of this term.
His main argument being that, under any interpretation known to him, the probability of a novel sentence must be zero, and since novel sentences are in fact generated all the time, there is a contradiction. The resolution of this contradiction is of course that it is not necessary to assign a probability of zero to a novel sentence; in fact, with current probabilistic models it is well-known how to assign a non-zero probability to novel occurrences, so this criticism is invalid, but was very influential for decades. Previously, in Syntactic Structures (1957) Chomsky wrote:
I think we are forced to conclude that ... probabilistic models give no particular insight into some of the basic problems of syntactic structure.In the footnote to this conclusion he considers the possibility of a useful probabilistic/statistical model, saying "I would certainly not care to argue that ... is unthinkable, but I know of no suggestion to this effect that does not have obvious flaws." The main "obvious flaw" is this: Consider:
- I never, ever, ever, ever, ... fiddle around in any way with electrical equipment.
- She never, ever, ever, ever, ... fiddles around in any way with electrical equipment.
- * I never, ever, ever, ever, ... fiddles around in any way with electrical equipment.
- * She never, ever, ever, ever, ... fiddle around in any way with electrical equipment.
This criticism is correct, but it is a criticism of Markov-chain models—it has nothing to do with probabilistic models (or trained models) at all. Moreover, since 1957 we have seen many types of probabilistic language models beyond the Markov-chain word models. Examples 1-4 above can in fact be distinguished with a finite-state model that is not a chain, but other examples require more sophisticated models. The best studied is probabilistic context-free grammar (PCFG), which operates over trees, categories of words, and individual lexical items, and has none of the restrictions of finite-state models. We find that PCFGs are state-of-the-art for parsing performance and are easier to learn from data than categorical context-free grammars. Other types of probabilistic models cover semantic and discourse structures. Every probabilistic model is a superset of a deterministic model (because the deterministic model could be seen as a probabilistic model where the probabilities are restricted to be 0 or 1), so any valid criticism of probabilistic models would have to be because they are too expressive, not because they are not expressive enough.
In Syntactic Structures, Chomsky introduces a now-famous example that is another criticism of finite-state probabilistic models:
Neither (a) 'colorless green ideas sleep furiously' nor (b) 'furiously sleep ideas green colorless', nor any of their parts, has ever occurred in the past linguistic experience of an English speaker. But (a) is grammatical, while (b) is not.Chomsky appears to be correct that neither sentence appeared in the published literature before 1955. I'm not sure what he meant by "any of their parts," but certainly every two-word part had occurred, for example:
- "It is neutral green, colorless green, like the glaucous water lying in a cellar." The Paris we remember, Elisabeth Finley Thomas (1942).
- "To specify those green ideas is hardly necessary, but you may observe Mr. [D. H.] Lawrence in the role of the satiated aesthete." The New Republic: Volume 29 p. 184, William White (1922).
- "Ideas sleep in books." Current Opinion: Volume 52, (1912).
But regardless of what is meant by "part," a statistically-trained finite-state model can in fact distinguish between these two sentences. Pereira (2001) showed that such a model, augmented with word categories and trained by expectation maximization on newspaper text, computes that (a) is 200,000 times more probable than (b). To prove that this was not the result of Chomsky's sentence itself sneaking into newspaper text, I repeated the experiment, using a much cruder model with Laplacian smoothing and no categories, trained over the Google Book corpus from 1800 to 1954, and found that (a) is about 10,000 times more probable. If we had a probabilistic model over trees as well as word sequences, we could perhaps do an even better job of computing degree of grammaticality.
Furthermore, the statistical models are capable of delivering the judgment that both sentences are extremely improbable, when compared to, say, "Effective green products sell well." Chomsky's theory, being categorical, cannot make this distinction; all it can distinguish is grammatical/ungrammatical.
Another part of Chomsky's objection is "we cannot seriously propose that a child learns the values of 109 parameters in a childhood lasting only 108 seconds." (Note that modern models are much larger than the 109 parameters that were contemplated in the 1960s.) But of course nobody is proposing that these parameters are learned one-by-one; the right way to do learning is to set large swaths of near-zero parameters simultaneously with a smoothing or regularization procedure, and update the high-probability parameters continuously as observations comes in. And noone is suggesting that Markov models by themselves are a serious model of human language performance. But I (and others) suggest that probabilistic, trained models are a better model of human language performance than are categorical, untrained models. And yes, it seems clear that an adult speaker of English does know billions of language facts (for example, that one says "big game" rather than "large game" when talking about an important football game). These facts must somehow be encoded in the brain.
It seems clear that probabilistic models are better for judging the likelihood of a sentence, or its degree of sensibility. But even if you are not interested in these factors and are only interested in the grammaticality of sentences, it still seems that probabilistic models do a better job at describing the linguistic facts. The mathematical theory of formal languages defines a language as a set of sentences. That is, every sentence is either grammatical or ungrammatical; there is no need for probability in this framework. But natural languages are not like that. A scientific theory of natural languages must account for the many phrases and sentences which leave a native speaker uncertain about their grammaticality (see Chris Manning's article and its discussion of the phrase "as least as"), and there are phrases which some speakers find perfectly grammatical, others perfectly ungrammatical, and still others will flip-flop from one occasion to the next. Finally, there are usages which are rare in a language, but cannot be dismissed if one is concerned with actual data. For example, the verb quake is listed as intransitive in dictionaries, meaning that (1) below is grammatical, and (2) is not, according to a categorical theory of grammar.
- The earth quaked.
- ? It quaked her bowels.
Steve Abney points out that probabilistic models are better suited for modeling language change. He cites the example of a 15th century Englishman who goes to the pub every day and orders "Ale!" Under a categorical model, you could reasonably expect that one day he would be served eel, because the great vowel shift flipped a Boolean parameter in his mind a day before it flipped the parameter in the publican's. In a probabilistic framework, there will be multiple parameters, perhaps with continuous values, and it is easy to see how the shift can take place gradually over two centuries.
Thus it seems that grammaticality is not a categorical, deterministic judgment but rather an inherently probabilistic one. This becomes clear to anyone who spends time making observations of a corpus of actual sentences, but can remain unknown to those who think that the object of study is their own set of intuitions about grammaticality. Both observation and intuition have been used in the history of science, so neither is "novel," but it is observation, not intuition that is the dominant model for science.
Now let's consider what I think is Chomsky's main point of disagreement with statistical models: the tension between "accurate description" and "insight." This is an old distinction. Charles Darwin (biologist, 1809–1882) is best known for his insightful theories but he stressed the importance of accurate description, saying "False facts are highly injurious to the progress of science, for they often endure long; but false views, if supported by some evidence, do little harm, for every one takes a salutary pleasure in proving their falseness." More recently, Richard Feynman (physicist, 1918–1988) wrote "Physics can progress without the proofs, but we can't go on without the facts."
On the other side, Ernest Rutherford (physicist, 1871–1937) disdained mere description, saying "All science is either physics or stamp collecting." Chomsky stands with him: "You can also collect butterflies and make many observations. If you like butterflies, that's fine; but such work must not be confounded with research, which is concerned to discover explanatory principles."
Acknowledging both sides is Robert Millikan (physicist, 1868–1953) who said in his Nobel acceptance speech "Science walks forward on two feet, namely theory and experiment ... Sometimes it is one foot that is put forward first, sometimes the other, but continuous progress is only made by the use of both."
The two cultures
After all those distinguished scientists have weighed in, I think the most relevant contribution to the current discussion is the 2001 paper by Leo Breiman (statistician, 1928–2005), Statistical Modeling: The Two Cultures. In this paper Breiman, alluding to C.P. Snow, describes two cultures:
First the data modeling culture (to which, Breiman estimates, 98% of statisticians subscribe) holds that nature can be described as a black box that has a relatively simple underlying model which maps from input variables to output variables (with perhaps some random noise thrown in). It is the job of the statistician to wisely choose an underlying model that reflects the reality of nature, and then use statistical data to estimate the parameters of the model.
Second the algorithmic modeling culture (subscribed to by 2% of statisticians and many researchers in biology, artificial intelligence, and other fields that deal with complex phenomena), which holds that nature's black box cannot necessarily be described by a simple model. Complex algorithmic approaches (such as support vector machines or boosted decision trees or deep belief networks) are used to estimate the function that maps from input to output variables, but we have no expectation that the form of the function that emerges from this complex algorithm reflects the true underlying nature.
It seems that the algorithmic modeling culture is what Chomsky is objecting to most vigorously. It is not just that the models are statistical (or probabilistic), it is that they produce a form that, while accurately modeling reality, is not easily interpretable by humans, and makes no claim to correspond to the generative process used by nature. In other words, algorithmic modeling describes what does happen, but it doesn't answer the question of why.
Breiman's article explains his objections to the first culture, data modeling. Basically, the conclusions made by data modeling are about the model, not about nature. (Aside: I remember in 2000 hearing James Martin, the leader of the Viking missions to Mars, saying that his job as a spacecraft engineer was not to land on Mars, but to land on the model of Mars provided by the geologists.) The problem is, if the model does not emulate nature well, then the conclusions may be wrong. For example, linear regression is one of the most powerful tools in the statistician's toolbox. Therefore, many analyses start out with "Assume the data are generated by a linear model..." and lack sufficient analysis of what happens if the data are not in fact generated that way. In addition, for complex problems there are usually many alternative good models, each with very similar measures of goodness of fit. How is the data modeler to choose between them? Something has to give. Breiman is inviting us to give up on the idea that we can uniquely model the true underlying form of nature's function from inputs to outputs. Instead he asks us to be satisfied with a function that accounts for the observed data well, and generalizes to new, previously unseen data well, but may be expressed in a complex mathematical form that may bear no relation to the "true" function's form (if such a true function even exists). Chomsky takes the opposite approach: he prefers to keep a simple, elegant model, and give up on the idea that the model will represent the data well. Instead, he declares that what he calls performance data—what people actually do—is off limits to linguistics; what really matters is competence—what he imagines that they should do.
In January of 2011, television personality Bill O'Reilly weighed in on more than one culture war with his statement "tide goes in, tide goes out. Never a miscommunication. You can't explain that," which he proposed as an argument for the existence of God. O'Reilly was ridiculed by his detractors for not knowing that tides can be readily explained by a system of partial differential equations describing the gravitational interaction of sun, earth, and moon (a fact that was first worked out by Laplace in 1776 and has been considerably refined since; when asked by Napoleon why the creator did not enter into his calculations, Laplace said "I had no need of that hypothesis."). (O'Reilly also seems not to know about Deimos and Phobos (two of my favorite moons in the entire solar system, along with Europa, Io, and Titan), nor that Mars and Venus orbit the sun, nor that the reason Venus has no moons is because it is so close to the sun that there is scant room for a stable lunar orbit.) But O'Reilly realizes that it doesn't matter what his detractors think of his astronomical ignorance, because his supporters think he has gotten exactly to the key issue: why? He doesn't care how the tides work, tell him why they work. Why is the moon at the right distance to provide a gentle tide, and exert a stabilizing effect on earth's axis of rotation, thus protecting life here? Why does gravity work the way it does? Why does anything at all exist rather than not exist? O'Reilly is correct that these questions can only be addressed by mythmaking, religion or philosophy, not by science.
Chomsky has a philosophy based on the idea that we should focus on the deep whys and that mere explanations of reality don't matter. In this, Chomsky is in complete agreement with O'Reilly. (I recognize that the previous sentence would have an extremely low probability in a probabilistic model trained on a newspaper or TV corpus.) Chomsky believes a theory of language should be simple and understandable, like a linear regression model where we know the underlying process is a straight line, and all we have to do is estimate the slope and intercept.
For example, consider the notion of a pro-drop language from Chomsky's Lectures on Government and Binding (1981). In English we say, for example, "I'm hungry," expressing the pronoun "I". But in Spanish, one expresses the same thought with "Tengo hambre" (literally "have hunger"), dropping the pronoun "Yo". Chomsky's theory is that there is a "pro-drop parameter" which is "true" in Spanish and "false" in English, and that once we discover the small set of parameters that describe all languages, and the values of those parameters for each language, we will have achieved true understanding.
The problem is that reality is messier than this theory. Here are some dropped pronouns in English:
- "Not gonna do it. Wouldn't be prudent." (Dana Carvey, impersonating George H. W. Bush)
- "Thinks he can outsmart us, does he?" (Evelyn Waugh, The Loved One)
- "Likes to fight, does he?" (S.M. Stirling, The Sunrise Lands)
- "Thinks he's all that." (Kate Brian, Lucky T)
- "Go for a walk?" (countless dog owners)
- "Gotcha!" "Found it!" "Looks good to me!" (common expressions)
From the beginning, Chomsky has focused on the generative side of language. From this side, it is reasonable to tell a non-probabilistic story: I know definitively the idea I want to express—I'm starting from a single semantic form—thus all I have to do is choose the words to say it; why can't that be a deterministic, categorical process? If Chomsky had focused on the other side, interpretation, as Claude Shannon did, he may have changed his tune. In interpretation (such as speech recognition) the listener receives a noisy, ambiguous signal and needs to decide which of many possible intended messages is most likely. Thus, it is obvious that this is inherently a probabilistic problem, as was recognized early on by all researchers in speech recognition, and by scientists in other fields that do interpretation: the astronomer Laplace said in 1819 "Probability theory is nothing more than common sense reduced to calculation," and the physicist James Maxwell said in 1850 "The true logic for this world is the calculus of Probabilities, which takes account of the magnitude of the probability which is, or ought to be, in a reasonable man's mind."
Finally, one more reason why Chomsky dislikes statistical models is that they tend to make linguistics an empirical science (a science about how people actually use language) rather than a mathematical science (an investigation of the mathematical properties of models of formal language). Chomsky prefers the later, as evidenced by his statement in Aspects of the Theory of Syntax (1965):
Linguistic theory is mentalistic, since it is concerned with discovering a mental reality underlying actual behavior. Observed use of language ... may provide evidence ... but surely cannot constitute the subject-matter of linguistics, if this is to be a serious discipline.I can't imagine Laplace saying that observations of the planets cannot constitute the subject-matter of orbital mechanics, or Maxwell saying that observations of electrical charge cannot constitute the subject-matter of electromagnetism. It is true that physics considers idealizations that are abstractions from the messy real world. For example, a class of mechanics problems ignores friction. But that doesn't mean that friction is not considered part of the subject-matter of physics.So how could Chomsky say that observations of language cannot be the subject-matter of linguistics? It seems to come from his viewpoint as a Platonist and a Rationalist and perhaps a bit of a Mystic. As in Plato's allegory of the cave, Chomsky thinks we should focus on the ideal, abstract forms that underlie language, not on the superficial manifestations of language that happen to be perceivable in the real world. That is why he is not interested in language performance. But Chomsky, like Plato, has to answer where these ideal forms come from. Chomsky (1991) shows that he is happy with a Mystical answer, although he shifts vocabulary from "soul" to "biological endowment."
Plato's answer was that the knowledge is 'remembered' from an earlier existence. The answer calls for a mechanism: perhaps the immortal soul ... rephrasing Plato's answer in terms more congenial to us today, we will say that the basic properties of cognitive systems are innate to the mind, part of human biological endowment.
It was reasonable for Plato to think that the ideal of, say, a horse, was more important than any individual horse we can perceive in the world. In 400BC, species were thought to be eternal and unchanging. We now know that is not true; that the horses on another cave wall—in Lascaux—are now extinct, and that current horses continue to evolve slowly over time. Thus there is no such thing as a single ideal eternal "horse" form.
We also now know that language is like that as well: languages are complex, random, contingent biological processes that are subject to the whims of evolution and cultural change. What constitutes a language is not an eternal ideal form, represented by the settings of a small number of parameters, but rather is the contingent outcome of complex processes. Since they are contingent, it seems they can only be analyzed with probabilistic models. Since people have to continually understand the uncertain. ambiguous, noisy speech of others, it seems they must be using something like probabilistic reasoning. Chomsky for some reason wants to avoid this, and therefore he must declare the actual facts of language use out of bounds and declare that true linguistics only exists in the mathematical realm, where he can impose the formalism he wants. Then, to get language from this abstract, eternal, mathematical realm into the heads of people, he must fabricate a mystical facility that is exactly tuned to the eternal realm. This may be very interesting from a mathematical point of view, but it misses the point about what language is, and how it works.
Thanks
Thanks to Ann Farmer, Fernando Pereira, Dan Jurafsky, Hal Varian, and others for comments and suggestions on this essay.Annotated Bibliography
- Abney, Steve (1996) Statistical Methods and Linguistics, in Klavans and Resnik (eds.) The Balancing Act: Combining Symbolic and Statistical Approaches to Language, MIT Press.
An excellent overall introduction to the statistical approach to language processing, and covers some ground that is not addressed often, such as language change and individual differences.
- Breiman, Leo (2001) Statistical Modeling: The Two Cultures, Statistical Science, Vol. 16, No. 3, 199-231.
Breiman does a great job of describing the two approaches, explaining the benefits of his approach, and defending his points in the vary interesting commentary with eminent statisticians: Cox, Efron, Hoadley, and Parzen.
- Chomsky, Noam (1956) Three Models for the Description of Language, IRE Transactions on Information theory (2), pp. 113-124.
Compares finite state, phrase structure, and transformational grammars. Introduces "colorless green ideas sleep furiously."
- Chomsky, Noam (1967) Syntactic Structures, Mouton.
A book-length exposition of Chomsky's theory that was the leading exposition of linguistics for a decade. Claims that probabilistic models give no insight into syntax.
- Chomsky, Noam (1969) Some Empirical Assumptions in Modern Philosophy of Language, in Philosophy, Science and Method: Essays in Honor or Ernest Nagel, St. Martin's Press.
Claims that the notion "probability of a sentence" is an entirely useless notion.
- Chomsky, Noam (1981) Lectures on government and binding, de Gruyer.
A revision of Chomsky's theory; this version introduces Universal Grammar. We cite it for the coverage of parameters such as pro-drop.
- Chomsky, Noam (1991) Linguistics and adjacent fields: a personal view, in Kasher (ed.), A Chomskyan Turn, Oxford.
I found the Plato quotes in this article, published by the Communist Party of Great Britain, and apparently published by someone with no linguistics training whatsoever, but with a political agenda.
- Gold, E. M. (1967) Language Identification in the Limit, Information and Control, Vol. 10, No. 5, pp. 447-474.
Gold proved a result in formal language theory that we can state (with some artistic license) as this: imagine a game between two players, guesser and chooser. Chooser says to guesser, "Here is an infinite number of languages. I'm going to choose one of them, and start reading sentences to you that come from that language. On your N-th birthday there will be a True-False quiz where I give you 100 sentences you haven't heard yet, and you have to say whether they come from the language or not." There are some limits on what the infinite set looks like and on how the chooser can pick sentences (he can be deliberately tricky, but he can't just repeat the same sentence over and over, for example). Gold's result is that if the infinite set of languages are all generated by context-free grammars then there is no strategy for guesser that guarantees she gets 100% correct every time, no matter what N you choose for the birthday. This result was taken by Chomsky and others to mean that it is impossible for children to learn human languages without having an innate "language organ." As Johnson (2004) and others show, this was an invalid conclusion; the task of getting 100% on the quiz (which Gold called language identification) really has nothing in common with the task of language acquisition performed by children, so Gold's Theorem has no relevance.
- Horning, J. J. (1969) A study of grammatical inference, Ph.D. thesis, Stanford Univ.
Where Gold found a negative result—that context-free languages were not identifiable from examples, Horning found a positive result—that probabilistic context-free languages are identifiable (to within an arbitrarily small level of error). Nobody doubts that humans have unique innate capabilities for understanding language (although it is unknown to what extent these capabilities are specific to language and to what extent they are general cognitive abilities related to sequencing and forming abstractions). But Horning proved in 1969 that Gold cannot be used as a convincing argument for an innate language organ that specifies all of language except for the setting of a few parameters.
- Johnson, Kent (2004) Gold's Theorem and cognitive science, Philosophy of Science, Vol. 71, pp. 571-592.
The best article I've seen on what Gold's Theorem actually says and what has been claimed about it (correctly and incorrectly). Concludes that Gold has something to say about formal languages, but nothing about child language acquisition.
- Lappin, Shalom and Shieber, Stuart M. (2007) Machine learning theory and practice as a source of insight into universal grammar., Journal of Linguistics, Vol. 43, No. 2, pp. 393-427.
An excellent article discussing the poverty of the stimulus, the fact that all models have bias, the difference between supervised and unsupervised learning, and modern (PAC or VC) learning theory. It provides alternatives to the model of Universal Grammar consisting of a fixed set of binary parameters.
- Manning, Christopher (2002) Probabilistic Syntax, in Bod, Hay, and Jannedy (eds.), Probabilistic Linguistics, MIT Press.
A compelling introduction to probabilistic syntax, and how it is a better model for linguistic facts than categorical syntax. Covers "the joys and perils of corpus linguistics."
- Norvig, Peter (2007) How to Write a Spelling Corrector, unpublished web page.
Shows working code to implement a probabilistic, statistical spelling correction algorithm.
- Norvig, Peter (2009) Natural Language Corpus Data, in Seagran and Hammerbacher (eds.), Beautiful Data, O'Reilly.
Expands on the essay above; shows how to implement three tasks: text segmentation, cryptographic decoding, and spelling correction (in a slightly more complete form than the previous essay).
- Pereira, Fernando (2002) Formal grammar and information theory: together again?, in Nevin and Johnson (eds.), The Legacy of Zellig Harris, Benjamins.
When I set out to write the page you are reading now, I was concentrating on the events that took place in Cambridge, Mass., 4800 km from home. After doing some research I was surprised to learn that the authors of two of the three best articles on this subject sit within a total of 10 meters from my desk: Fernando Pereira and Chris Manning. (The third, Steve Abney, sits 3700 km away.) But perhaps I shouldn't have been surprised. I remember giving a talk at ACL on the corpus-based language models used at Google, and having Fernando, then a professor at U. Penn., comment "I feel like I'm a particle physicist and you've got the only super-collider." A few years later he moved to Google. Fernando is also famous for his quote "The older I get, the further down the Chomsky Hierarchy I go." His article here covers some of the same ground as mine, but he goes farther in explaining the range of probabilistic models available and how they are useful.
- Plato (c. 380BC) The Republic.
Cited here for the allegory of the cave.
- Shannon, C.E. (1948) A Mathematical Theory of Communication, The Bell System Technical Journal, Vol. 27, pp. 379-423.
An enormously influential article that started the field of information theory and introduced the term "bit" and the noisy channel model, demonstrated successive n-gram approximations of English, described Markov models of language, defined entropy with respect to these models, and enabled the growth of the telecommunications industry.
[3] End of Theory.
THE END OF THEORY: THE DATA DELUGE MAKES THE SCIENTIFIC METHOD OBSOLETE

Illustration: Marian Bantjes "All models are wrong, but some are useful."
So proclaimed statistician George Box 30 years ago, and he was right. But what choice did we have? Only models, from cosmological equations to theories of human behavior, seemed to be able to consistently, if imperfectly, explain the world around us. Until now. Today companies like Google, which have grown up in an era of massively abundant data, don't have to settle for wrong models. Indeed, they don't have to settle for models at all.
Sixty years ago, digital computers made information readable. Twenty years ago, the Internet made it reachable. Ten years ago, the first search engine crawlers made it a single database. Now Google and like-minded companies are sifting through the most measured age in history, treating this massive corpus as a laboratory of the human condition. They are the children of the Petabyte Age.
The Petabyte Age is different because more is different. Kilobytes were stored on floppy disks. Megabytes were stored on hard disks. Terabytes were stored in disk arrays. Petabytes are stored in the cloud. As we moved along that progression, we went from the folder analogy to the file cabinet analogy to the library analogy to — well, at petabytes we ran out of organizational analogies.
At the petabyte scale, information is not a matter of simple three- and four-dimensional taxonomy and order but of dimensionally agnostic statistics. It calls for an entirely different approach, one that requires us to lose the tether of data as something that can be visualized in its totality. It forces us to view data mathematically first and establish a context for it later. For instance, Google conquered the advertising world with nothing more than applied mathematics. It didn't pretend to know anything about the culture and conventions of advertising — it just assumed that better data, with better analytical tools, would win the day. And Google was right.
Google's founding philosophy is that we don't know why this page is better than that one: If the statistics of incoming links say it is, that's good enough. No semantic or causal analysis is required. That's why Google can translate languages without actually "knowing" them (given equal corpus data, Google can translate Klingon into Farsi as easily as it can translate French into German). And why it can match ads to content without any knowledge or assumptions about the ads or the content.
Speaking at the O'Reilly Emerging Technology Conference this past March, Peter Norvig, Google's research director, offered an update to George Box's maxim: "All models are wrong, and increasingly you can succeed without them."
This is a world where massive amounts of data and applied mathematics replace every other tool that might be brought to bear. Out with every theory of human behavior, from linguistics to sociology. Forget taxonomy, ontology, and psychology. Who knows why people do what they do? The point is they do it, and we can track and measure it with unprecedented fidelity. With enough data, the numbers speak for themselves.
The big target here isn't advertising, though. It's science. The scientific method is built around testable hypotheses. These models, for the most part, are systems visualized in the minds of scientists. The models are then tested, and experiments confirm or falsify theoretical models of how the world works. This is the way science has worked for hundreds of years.
Scientists are trained to recognize that correlation is not causation, that no conclusions should be drawn simply on the basis of correlation between X and Y (it could just be a coincidence). Instead, you must understand the underlying mechanisms that connect the two. Once you have a model, you can connect the data sets with confidence. Data without a model is just noise.
But faced with massive data, this approach to science — hypothesize, model, test — is becoming obsolete. Consider physics: Newtonian models were crude approximations of the truth (wrong at the atomic level, but still useful). A hundred years ago, statistically based quantum mechanics offered a better picture — but quantum mechanics is yet another model, and as such it, too, is flawed, no doubt a caricature of a more complex underlying reality. The reason physics has drifted into theoretical speculation about n-dimensional grand unified models over the past few decades (the "beautiful story" phase of a discipline starved of data) is that we don't know how to run the experiments that would falsify the hypotheses — the energies are too high, the accelerators too expensive, and so on.
Now biology is heading in the same direction. The models we were taught in school about "dominant" and "recessive" genes steering a strictly Mendelian process have turned out to be an even greater simplification of reality than Newton's laws. The discovery of gene-protein interactions and other aspects of epigenetics has challenged the view of DNA as destiny and even introduced evidence that environment can influence inheritable traits, something once considered a genetic impossibility.
In short, the more we learn about biology, the further we find ourselves from a model that can explain it.
There is now a better way. Petabytes allow us to say: "Correlation is enough." We can stop looking for models. We can analyze the data without hypotheses about what it might show. We can throw the numbers into the biggest computing clusters the world has ever seen and let statistical algorithms find patterns where science cannot.
The best practical example of this is the shotgun gene sequencing by J. Craig Venter. Enabled by high-speed sequencers and supercomputers that statistically analyze the data they produce, Venter went from sequencing individual organisms to sequencing entire ecosystems. In 2003, he started sequencing much of the ocean, retracing the voyage of Captain Cook. And in 2005 he started sequencing the air. In the process, he discovered thousands of previously unknown species of bacteria and other life-forms.
If the words "discover a new species" call to mind Darwin and drawings of finches, you may be stuck in the old way of doing science. Venter can tell you almost nothing about the species he found. He doesn't know what they look like, how they live, or much of anything else about their morphology. He doesn't even have their entire genome. All he has is a statistical blip — a unique sequence that, being unlike any other sequence in the database, must represent a new species.
This sequence may correlate with other sequences that resemble those of species we do know more about. In that case, Venter can make some guesses about the animals — that they convert sunlight into energy in a particular way, or that they descended from a common ancestor. But besides that, he has no better model of this species than Google has of your MySpace page. It's just data. By analyzing it with Google-quality computing resources, though, Venter has advanced biology more than anyone else of his generation.
This kind of thinking is poised to go mainstream. In February, the National Science Foundation announced the Cluster Exploratory, a program that funds research designed to run on a large-scale distributed computing platform developed by Google and IBM in conjunction with six pilot universities. The cluster will consist of 1,600 processors, several terabytes of memory, and hundreds of terabytes of storage, along with the software, including IBM's Tivoli and open source versions of Google File System and MapReduce.11 Early CluE projects will include simulations of the brain and the nervous system and other biological research that lies somewhere between wetware and software.
Learning to use a "computer" of this scale may be challenging. But the opportunity is great: The new availability of huge amounts of data, along with the statistical tools to crunch these numbers, offers a whole new way of understanding the world. Correlation supersedes causation, and science can advance even without coherent models, unified theories, or really any mechanistic explanation at all.
There's no reason to cling to our old ways. It's time to ask: What can science learn from Google?
Chris Anderson (canderson@wired.com) is the editor in chief of Wired.
Thankyou for such useful article. Grow your career as a data scientist with Data Science Course in Greater Noida.
ReplyDelete